In today’s fast-paced business environment, accurately forecasting market trends is vital for making informed decisions. The dynamic nature of markets—shaped by shifting consumer preferences, global economic conditions, and technological advancements—demands sophisticated tools for data analysis. Building robust data models that can adapt to these changes is key to achieving long-term business success.
A crucial aspect of mastering market forecasting lies in obtaining the right data, analysing it efficiently, and using advanced statistical techniques and machine learning algorithms. By applying these methods, businesses can gain valuable insights into future market conditions. For individuals interested in harnessing the power of data analytics for such tasks, a data science course in Pune can provide the foundational and advanced skills required to build these models.
Importance of Market Forecasting
Market forecasting helps businesses predict how key factors such as demand, supply, and pricing will move. With a comprehensive understanding of market trends, companies can optimise inventory, pricing, and product development strategies. The complexity and volatility of the market demand accurate and flexible data models to account for sudden shifts in external conditions. This is where the role of data science comes in. By developing robust data models, businesses can better anticipate market trends, prepare for uncertainties, and align their strategies accordingly.
To excel in this field, a data scientist course can equip you with the analytical tools and techniques to handle large datasets, explore various predictive models, and apply machine learning algorithms for enhanced forecasting accuracy.
Key Components of Robust Data Models
Data Collection and Cleaning
The foundation of any reliable data model is high-quality data. Collecting accurate, relevant, and up-to-date data is crucial for effective market forecasting. This data can come from various sources, such as consumer surveys, social media interactions, sales history, and economic reports. However, raw data often contains inconsistencies, missing values, and noise that need to be cleaned before it can be analysed.
Data cleaning involves handling missing values, removing outliers, and ensuring consistency across different datasets. By preparing clean data, businesses can avoid the pitfalls of inaccurate or misleading predictions. Collecting and cleaning data is an essential skill covered extensively in a data scientist course.
Exploratory Data Analysis (EDA)
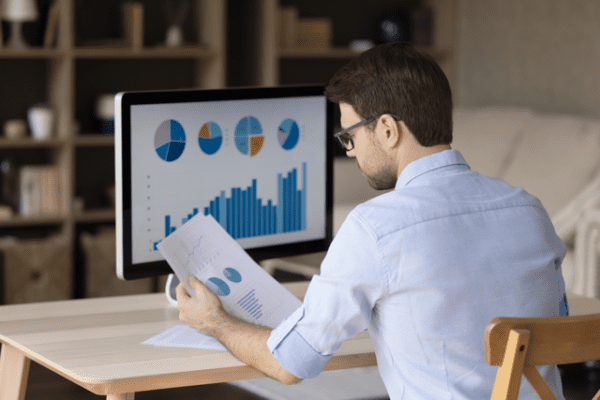
Once the data is prepared, it’s time for exploratory data analysis (EDA). EDA involves visually and statistically examining data to uncover underlying patterns, correlations, and trends. Analysts can identify key relationships between variables through techniques like histograms, scatter plots, and correlation matrices.
The insights gained from EDA allow businesses to choose the most relevant features for their forecasting models. A strong understanding of EDA is essential for anyone aiming to build reliable data models for market forecasting. A data scientist course teaches the techniques for conducting thorough exploratory analysis and identifying the right features for predictive modelling.
Choosing the Right Forecasting Models
Selecting the right forecasting model is critical to building an accurate data model. Several approaches are available, including statistical methods, machine learning algorithms, and hybrid models that combine multiple techniques. Some of the most common models used for market forecasting include:
- Linear Regression: A foundational statistical method to model the relationship between dependent and independent variables. It works well for predicting continuous variables based on linear relationships.
- Time Series Analysis: A statistical technique used to analyse data points ordered in time. It is particularly useful in forecasting future trends based on past data and includes models like ARIMA (AutoRegressive Integrated Moving Average).
- Machine Learning Algorithms: Algorithms such as decision trees, random forests, and support vector machines can provide more sophisticated and flexible solutions for forecasting. These algorithms can handle non-linear relationships and adapt to complex datasets.
Each forecasting model has its strengths and weaknesses, and choosing the right one depends on the nature of the data and the specific forecasting goals. By learning various modelling techniques through a data science course in Pune, aspiring data scientists can make informed decisions about which models are best suited for dynamic market forecasting.
Feature Engineering
Feature engineering is the process of selecting, modifying, or creating new variables (features) from raw data to improve the performance of predictive models. Good feature engineering can significantly enhance a model’s predictive power by highlighting important trends and relationships.
For instance, creating new features like moving averages, rolling windows, or lag variables can provide insights into time-dependent trends. Effective feature engineering requires domain knowledge and technical expertise, both of which are taught in a data science course in Pune.
Model Training and Validation
Once a suitable model is chosen and the features are engineered, the next step is training the model on historical data. During training, the model learns the patterns and relationships in the data for forecasting. However, the model’s performance should not be judged solely on training data. It is essential to validate the model on unseen data to evaluate its generalisation ability.
Validation techniques like cross-validation and using a separate test set help ensure that the model performs well in real-world scenarios, not just on the data it was trained on. A data science course in Pune covers the theory and practical application of model validation techniques to ensure that the developed models are robust and reliable.
Model Evaluation and Optimisation
After validation, the model’s performance must be evaluated using metrics such as Mean Absolute Error (MAE), Root Mean Squared Error (RMSE), and R-squared. These metrics provide insights into how well the model predicts future market trends.
Based on the evaluation, the model can be fine-tuned and optimised by adjusting hyperparameters, adding or removing features, or even trying alternative models. Iterative optimisation is key in building robust data models that can effectively forecast market trends. A data science course in Pune teaches learners how to evaluate and optimise their models for better performance.
Adapting to Market Changes
The market is inherently volatile, and forecasting models must be able to adapt to sudden changes in trends. This is where machine learning models that can learn and update themselves with new data come into play. Techniques like online and reinforcement learning allow models to evolve continuously, keeping them relevant in a constantly changing market.
By applying these advanced techniques, businesses can ensure that their forecasting models remain accurate. A data science course in Pune also covers adaptive models, providing learners with the skills to create data models that are not just robust but also flexible enough to handle market dynamics.
Conclusion
Building robust data models for dynamic market forecasting requires accurate data collection, advanced modelling techniques, and continuous optimisation. Businesses can create predictive models that provide valuable insights into future market conditions by understanding the importance of data preparation, exploratory analysis, model selection, and validation.
For those looking to develop these skills, a data science course in pune offers comprehensive training that combines theory and practical application. It equips learners with the tools they need to build effective data models for dynamic market forecasting. With the right approach and training, businesses can leverage data science to stay ahead of the competition and make well-informed decisions in an ever-changing market.
Business Name: ExcelR – Data Science, Data Analytics Course Training in Pune
Address: 101 A,1st Floor, Siddh Icon, Baner Rd, opposite Lane To Royal Enfield Showroom, beside Asian Box Restaurant, Baner, Pune, Maharashtra 411045
Phone Number: 098809 13504
Email : enquiry@excelr.com